Is a South Park Stock Market trading strategy viable?
In the following, we will use Python to get a list of all the mentions of public companies mentioned in South Park episodes. We’ll scrape Wikipedia for this purpose.
Following this, we’ll try to use this “alt-data” to generate a South Park Stock Market trading strategy.
Step 1: Get a list of all the individual episode wikis
We can see that the full list of episodes is available on this page, split between a few different tables, over here: https://en.wikipedia.org/wiki/List_of_South_Park_episodes.
While manually taking every link is an option, it’s an excruciating and time-consuming one. Luckily, we do have a better way of doing this!
Using the requests library, alongside BeautifulSoup, we can extract all the info on the URL:
url = 'https://en.wikipedia.org/wiki/List_of_South_Park_episodes' page = requests.get(url) soup = BeautifulSoup(page.content)
However, our “soup” contains a lot more than we need, so let’s try and find our specific info.
Looking at the page source, we notice that our desired information is situated in blocks with the following specifics:
table_class = "wikitable plainrowheaders wikiepisodetable" span_class = "bday dtstart published updated"
Using the information above, and a bit more tweaking, we arrive at a final code that loops through the tables in the page that have the appropriate class, and through their rows. The information is then gathered in a dictionary.
dict_sp={} i=1 for table in soup.find_all("table", class_=table_class): for tr in table.find_all('tr', class_="vevent"): if 'id' in tr.find('th').attrs.keys(): dict_sp[i]={'date':tr.find('span',class_=span_class).contents[0], 'episode_id':tr.find('th').attrs['id'], 'episode_wiki_link':tr.find('a', href=True, title=True).attrs['href'], 'episode_title':tr.find('a', href=True, title=True).attrs['title']} i=i+1
An entry in our resulting dict looks like this:
{'date': '1997-08-13', 'episode_id': 'ep1', 'episode_wiki_link': '/wiki/Cartman_Gets_an_Anal_Probe', 'episode_title': 'Cartman Gets an Anal Probe'}
Step 2: Crawl through each episode wiki and find all the wiki links in the pages
We first define a helper function:
def find_wiki_hrefs_in_soup(soup): wiki_hrefs=[] for elem in soup.find_all("a", href=True, title=True): elem_href=elem.attrs['href'] if elem_href.split("/")[1]=='wiki': wiki_hrefs.append(elem_href) return np.unique(wiki_hrefs)
Using it, we go through each item of our dictionary, apply the function, and append the new info to our dictionary. We also add a small sleep in between calls, so that we don’t overwhelm our environment and also so that we don’t get blocked by Wikipedia.
for key in dict_sp.keys(): url_ep = 'https://en.wikipedia.org/'+dict_sp[key]['episode_wiki_link'] page_ep = requests.get(url_ep) soup_ep = BeautifulSoup(page_ep.content) dict_sp[key]['links']=find_wiki_hrefs_in_soup(soup_ep) time.sleep(0.1)
Now, are dictionary looks like this (when converted to a Pandas DataFrame):
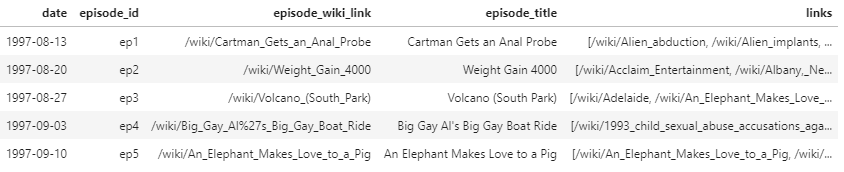
Step 3: See if any of the links match public companies
While the most straightforward approach would be to start crawling through all the links in all the episodes, this would not be ideal, as a lot of the links are repeated (i.e. almost all pages include a link to https://en.wikipedia.org/wiki/South_Park ). Thus, for the sake of efficiency, we first generate a list of all unique URLs:
all_urls=[] for key in dict_sp.keys(): current_urls=dict_sp[key]['links'] all_urls=np.unique([*all_urls, *current_urls])
For this list, we can start crawling. We notice that for all the public companies, Wikipedia has a VCard, containing a link to https://en.wikipedia.org/wiki/Public_company, but also the ticker. We’ll use this to check if our links refer to a public company, as well as to get the corresponding ticker. Using the page source, we find the class of the vcard table and define it as below, as well as the required match to the Public Company URL.
vcard_class = "infobox vcard" key_match = '/wiki/Public_company'
In a new dictionary, we will collect all the vcard information for all of our 10k + URLs (if they have one!).
dict_url = {} err = [] for wiki_url in all_urls: url = 'https://en.wikipedia.org'+wiki_url page = requests.get(url) soup = BeautifulSoup(page.content) try: dict_url[url]=find_wiki_hrefs_in_soup(soup.find("table",class_=vcard_class)) except: err.append("No vcard for "+ wiki_url) time.sleep(0.1)
We end up with a list of 49 URLs, but some manual tinkering is required for the tickers, due to the very different formats used in the vcards, differences in ticker name across regions, over-the-counter securities, as well as for duplicate pages such as the below:
'https://en.wikipedia.org/wiki/ViacomCBS', 'https://en.wikipedia.org/wiki/Viacom_(2005%E2%80%93present)',
Final Results
Any South Park fan could name a few examples of situations in which Public Companies were mentioned in South Park episodes. For me, these would be the most table three examples:
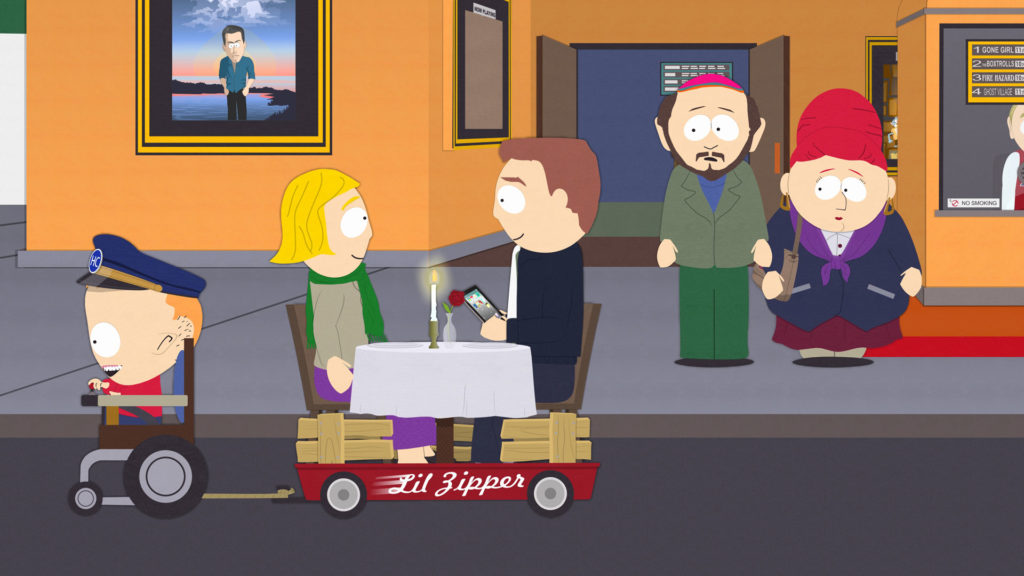
Handicar – Uber, Lyft, and Tesla
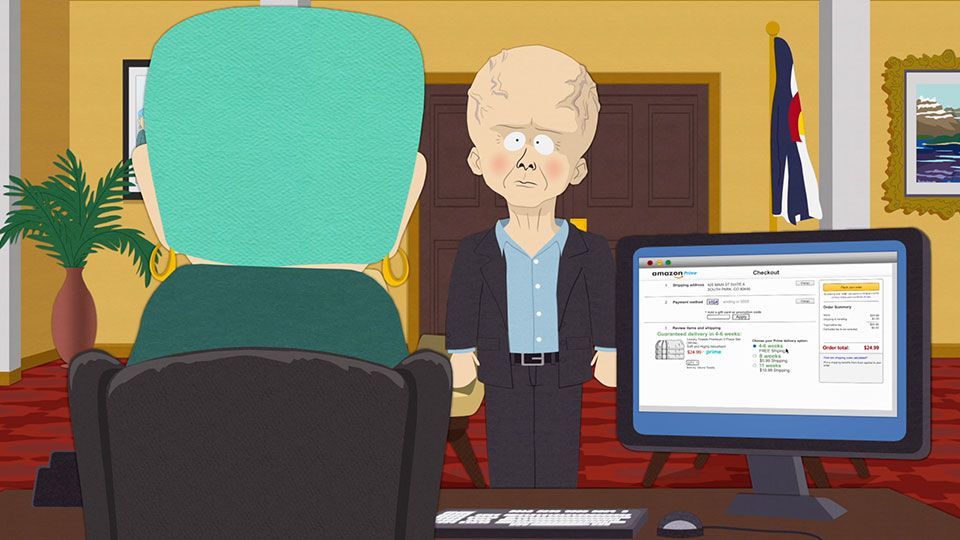
End notes
We’ve managed to meet our current goal, getting all the data that we needed for developing a strategy, in a fairly quick and simple way, thanks to “Requests” and “BeautifulSoup”.
If you’re also interested in how this can be used further for developing the “South Park Stock Market” trading strategy and whether or not it performs better than the SP500 (Standard&Poor) index fund, jump over to this post.